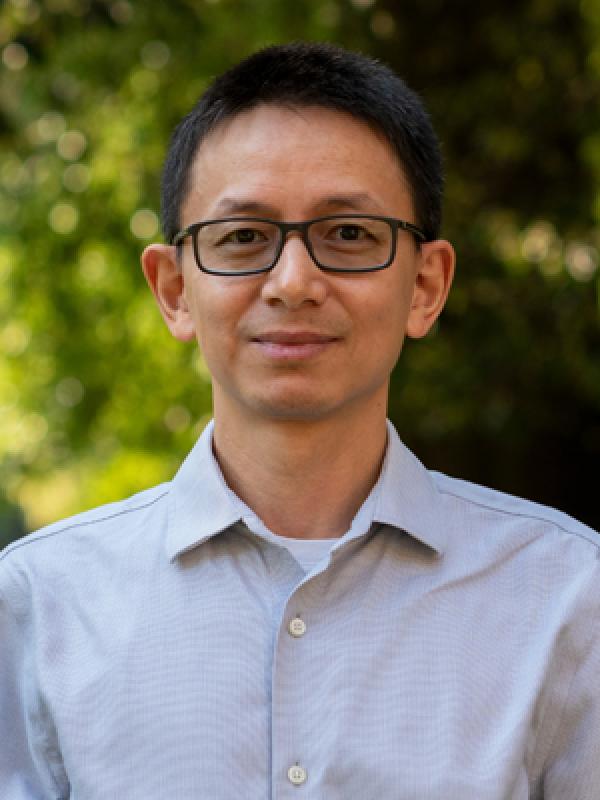
Desheng Liu
Professor
1056 Derby Hall
154 North Oval Mall
Columbus OH 43210
Areas of Expertise
- Remote Sensing
- Spatial Statistics
- GIScience
- Land Cover Change
Education
- Ph.D., 2006 Environmental Science, University of California, Berkeley
- M.A., 2004 Statistics, University of California, Berkeley
- M.S., 2003 Environmental Science, University of California, Berkeley
- B.E., 2001 GIS, Wuhan University, China
Current CV:
Interests: Remote Sensing, Spatial Statistics, GIScience, Land Cover Change.
Current Research: My research focuses on developing geo-spatial data analysis methodologies for monitoring and modeling environmental and ecological processes. It draws upon an array of geo-spatial and statistical approaches, particularly remote sensing and spatial statistics. While I have brought these approaches to bear on numerous research problems, a central theme in my research is the statistical modeling of the spatial or spatial-temporal dimension of the processes under study.
Courses Taught:
Geography 5100-Quantitative Geographical Methods
Geography 5270-Geographic Applications of Remote Sensing
Geography 8102-Application of Quantitative Methods in Geography
Statistics 6530-Introduction to Spatial Statistics
Select Publications:
Liu, D. and S. Cai. 2012. A spatial-temporal modeling approach to reconstructing land-cover change trajectories from multi-temporal satellite imagery. Annals of the Association of American Geographers (DOI: 10.1080/00045608.2011.596357).
Liu, D. and X. Zhu. 2012. An enhanced physical method for downscaling thermal infrared radiance. IEEE Geoscience and Remote Sensing Letters 9(4): 690-694.
Liu, D. and F. Xia. 2010. Assessing object-based classification: advantages and limitations. Remote Sensing Letters 1(4): 187-194.
Kang, E.L., D. Liu, and N. Cressie. 2009. Statistical analysis of small-area data based on independence, spatial, non-hierarchical, and hierarchical models. Computational Statistics and Data Analysis 53: 3016-3032.
Liu, D., K. Song, J.R. Townshend, and P. Gong. 2008. Using local transition probability models in Markov random fields for forest change detection. Remote Sensing of Environment 112(5): 2222-2231.